Data analysis and visualization are crucial components of decision-making processes in various fields, including business, science, and technology. As the volume of data continues to grow exponentially, finding innovative ways to make sense of this information becomes increasingly important. Generative Artificial Intelligence (AI) is emerging as a powerful tool in the realm of data analysis and visualization. In this blog, we will explore how generative AI can be leveraged to transform raw data into actionable insights and compelling visualizations.
Understanding Generative AI
Generative AI refers to a class of artificial intelligence algorithms designed to generate new content or data based on patterns and information from existing data. It operates on a variety of models, such as Generative Adversarial Networks (GANs) and Recurrent Neural Networks (RNNs), to create data that is similar to, yet distinct from, the training data.
One of the standout features of generative AI is its ability to generate data, text, images, or even sounds. In the context of data analysis and visualization, this capability is particularly valuable because it enables the creation of synthetic datasets, the generation of additional data points, and the development of innovative data visualizations.
Data Augmentation
Generative AI can be used to augment existing datasets by generating synthetic data points. This is especially useful in situations where obtaining more real-world data is costly or time-consuming. For instance, in medical research, where gathering patient data can be challenging due to privacy and ethical concerns, generative AI can be employed to create synthetic patient records that maintain the statistical characteristics of the original dataset. These synthetic records can then be used to expand the sample size and improve the accuracy of research findings.
Furthermore, in machine learning, having a larger dataset often leads to better model performance. By using generative AI to increase the size of a training dataset, you can enhance the accuracy and robustness of machine learning models, resulting in more reliable predictions.
Anomaly Detection
Generative AI is also invaluable in anomaly detection, where it can help identify irregularities and deviations in datasets. The generative model learns the normal patterns within the data, and when presented with a new data point that doesn’t conform to these patterns, it can flag it as an anomaly. This is particularly useful in fraud detection, network security, and quality control processes.
For instance, in financial transactions, generative AI can be used to model the regular spending behavior of an individual. When an unusual transaction occurs, the model can quickly detect it as an anomaly, helping to prevent fraud. In manufacturing, generative AI can identify defective products by recognizing deviations from normal quality parameters, leading to improved quality control.
Data Visualization
The power of generative AI extends beyond data analysis and manipulation. It can also be harnessed for creating innovative data visualizations. Traditional charts and graphs are often limited in their capacity to represent complex data structures. Generative AI can assist in developing novel visualization techniques that provide a deeper understanding of the data.
For example, generative AI can create visual representations of high-dimensional datasets in 2D or 3D space, making it easier for analysts to explore and identify patterns. It can generate heatmaps, network diagrams, or even artistic representations of data, adding a new dimension to data communication. These advanced visualizations can help users uncover insights that might be missed with traditional chart types.
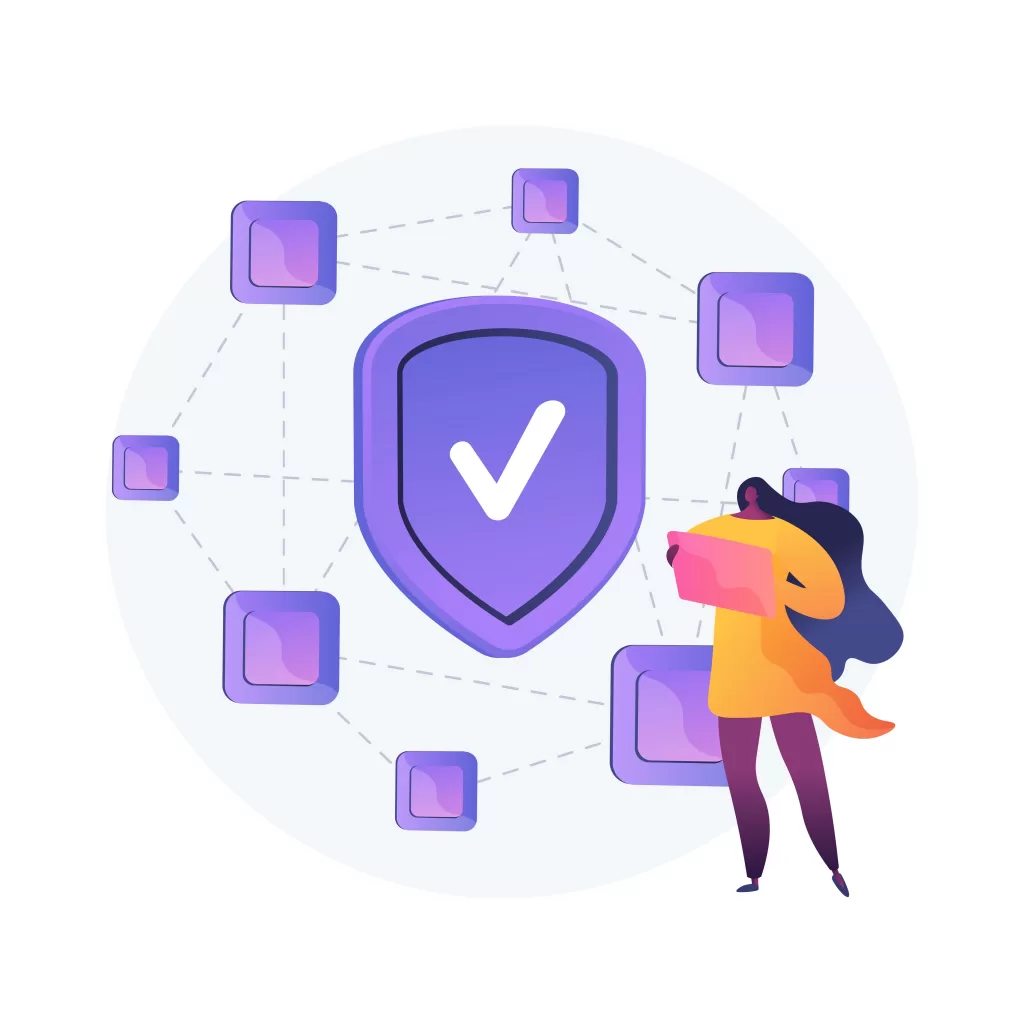
Challenges and Considerations
While generative AI offers numerous benefits for data analysis and visualization, it also presents challenges and considerations. Here are some important points to keep in mind:
Quality of Generated Data:
Data Fidelity:
The quality of data generated by AI models largely depends on the quality of the training data. If the training data is biased, noisy, or incomplete, the generated data can inherit these issues. Biased data can lead to biased generative models, potentially reinforcing and propagating existing biases.Overfitting:
Generative AI models can sometimes overfit to the training data, resulting in synthetic data that is too similar to the original dataset. This can limit the model’s ability to explore new patterns in the data.Data Variability:
In many real-world scenarios, data is highly variable and complex. Generative models may struggle to capture all the intricacies of such data, leading to simplified or unrealistic synthetic data.
Ethical Concerns:
Privacy:
Generating synthetic data can inadvertently reveal sensitive or private information. Even though the data is synthetic, it may still resemble real data closely enough to compromise individual privacy. Organizations must be cautious and consider privacy implications when using generative AI.Security:
In some cases, the use of generative AI for data analysis can lead to security risks. For instance, malicious actors could exploit generative models to create fake identities or documents for fraudulent purposes.
Interpretability:
Complex Models:
Many generative AI models, such as deep neural networks, are highly complex and operate as “black boxes.” Understanding how these models generate data can be challenging, making it difficult to validate the accuracy and reliability of the generated data.Model Understanding:
Lack of interpretability may hinder an organization’s ability to explain the decision-making process and justify the use of generative AI-generated data, particularly in regulated industries.
Computational Resources:
High Computational Demands:
Training and using generative AI models can be computationally intensive, requiring powerful hardware and significant processing time. Smaller organizations or researchers with limited computational resources may find this challenging.Energy Consumption:
Running complex models on large datasets can have a considerable environmental impact due to high energy consumption. This poses sustainability and cost concerns.
Regulatory Compliance:
Data Regulations:
Different industries and regions have specific data regulations, such as GDPR in Europe or HIPAA in the healthcare sector. Ensuring that the generated data complies with these regulations can be a complex task, particularly if the model lacks transparency.Data Validation:
Regulatory bodies may require organizations to validate and justify the use of generative AI-generated data. The lack of clear methods for validating synthetic data may pose compliance challenges.
Data Overgeneration:
Overproducing Data:
Generative AI models can sometimes overgenerate data, resulting in a flood of synthetic information that can overwhelm the analysis process. Managing and sifting through this surplus data can be a challenge.
Maintenance and Upkeep:
Model Drift:
Generative AI models can degrade in performance over time if not regularly retrained. This means that organizations need to allocate resources for continuous model maintenance and updates.Hardware and Software Updates:
As hardware and software evolve, maintaining compatibility with existing generative models can become an issue, necessitating ongoing updates.
Validation and Trust:
Model Validation:
Trusting the results generated by generative models is a significant challenge. Ensuring that the synthetic data accurately represents the underlying reality is not always straightforward.Transparency and Trust:
Demonstrating that the generative model is fair, unbiased, and robust requires transparency in model development and continuous monitoring.
Educational and Skill Gaps:
Skills and Expertise:
Leveraging generative AI for data analysis and visualization requires a highly specialized skill set. Finding experts who can work with these technologies can be a challenge for organizations.
Costs:
Initial Investment:
Implementing generative AI can be costly, involving expenses for model development, computational resources, and staff training. Smaller organizations may find it challenging to justify these upfront costs.
Long-Term Viability:
Model Obsolescence:
AI models can become outdated relatively quickly in the fast-paced world of AI research. Organizations must consider the long-term viability of their generative AI solutions.
Conclusion
Generative AI is revolutionizing the way data is analyzed and visualized. It provides opportunities to expand datasets, detect anomalies, and create innovative visualizations. By harnessing the power of generative AI, organizations can unlock valuable insights and make data-driven decisions with greater confidence. However, it is essential to approach this technology with responsibility, ethics, and a clear understanding of the challenges it may pose. As generative AI continues to advance, its role in data analysis and visualization will undoubtedly become even more critical, shaping the future of decision-making processes in various fields.
Also Read: “Generative AI and the future of work in America“.
Add Comment