In the digital age, data has emerged as one of the most valuable assets for organizations across industries. The ability to collect, analyze, and leverage data has become a competitive advantage, enabling companies to make informed decisions, enhance customer experiences, and drive innovation. As organizations strive for digital transformation, data-driven decision making is at the forefront of their strategies. In this blog, we’ll explore how Generative AI is becoming a key pillar in this process, revolutionizing the way businesses harness data for their benefit.
The Power of Data-Driven Decision Making
Data-driven decision making is the process of using data analytics to guide business strategies and actions. It involves collecting, processing, and analyzing data to gain valuable insights, enabling organizations to make informed choices rather than relying on intuition or guesswork. This approach has several benefits, including:
Improved Decision Quality:
Data-driven decisions are based on facts and evidence, reducing the chances of making costly mistakes or errors in judgment. This precision in decision-making extends to various aspects of an organization:
Risk Mitigation:
Data-driven decision making helps organizations identify and assess risks more accurately. By analyzing historical data and market trends, businesses can proactively identify potential risks and develop strategies to mitigate them. This not only safeguards the organization’s financial health but also enhances its reputation and trustworthiness among stakeholders.
Strategic Planning:
In the realm of strategic planning, data-driven insights provide a solid foundation for setting long-term goals and objectives. By analyzing historical performance data and market dynamics, organizations can make strategic decisions that align with their vision and mission. This ensures that every step taken is in sync with the overarching goals, optimizing resource allocation and minimizing deviations from the strategic path.
Resource Optimization:
Data-driven decision making extends beyond immediate efficiency gains. It also involves optimizing resource allocation over time. By continuously analyzing data, organizations can adapt and reallocate resources as needed. For instance, in manufacturing, predictive maintenance based on data analysis can reduce downtime and improve resource allocation for maintenance teams.
Compliance and Governance:
In highly regulated industries, data-driven decisions play a crucial role in ensuring compliance with industry standards and government regulations. Organizations can use data to monitor and report on various compliance aspects, reducing the risk of legal and financial consequences.
Enhanced Efficiency:
By automating data collection and analysis, businesses can streamline processes and allocate resources more efficiently. This efficiency translates into various operational advantages:
Faster Decision-Making:
Automation speeds up the data-to-insights process, enabling organizations to make decisions faster. In industries like finance, where timely decisions are critical, automation can mean the difference between seizing or missing out on opportunities.
Resource Allocation:
Efficiency gains from data-driven automation allow organizations to allocate human resources more strategically. Employees can focus on higher-value tasks that require creativity, critical thinking, and human expertise, while routine tasks can be handled by automated systems.
Cost Reduction:
Streamlining processes through automation often leads to cost savings. Organizations can reduce manual labor, minimize errors, and optimize resource utilization, resulting in a more cost-effective operation.
Customer Service:
Automation in customer service, driven by data, can lead to faster response times, improved issue resolution, and better customer experiences. Chatbots and AI-driven support systems can handle routine inquiries, freeing up human agents to address more complex issues.
Supply Chain Optimization:
Data-driven insights can optimize supply chain operations, reducing lead times, minimizing inventory holding costs, and improving overall supply chain efficiency. This is particularly vital in industries where supply chain disruptions can have far-reaching consequences.
Generative AI: A Game-Changer in Data-Driven Decision Making
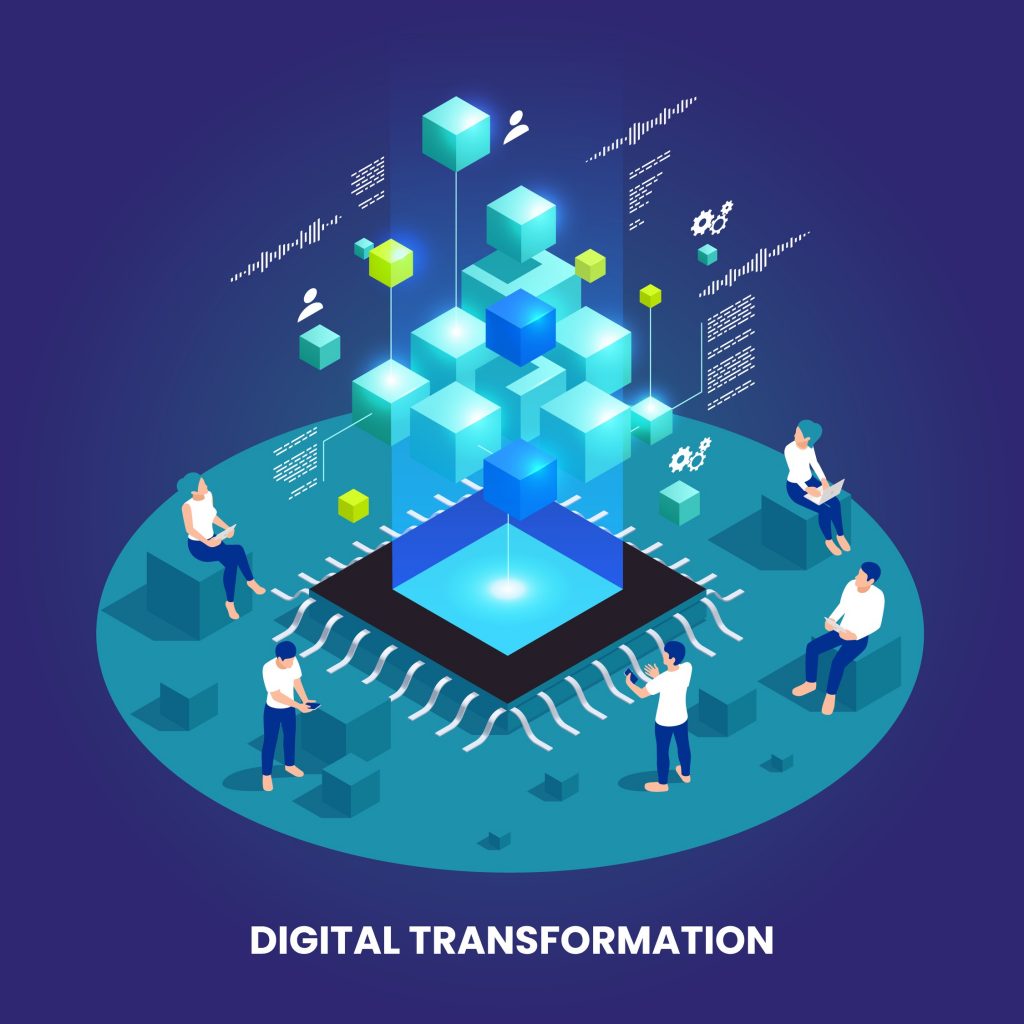
This subfield of AI, known as Generative Artificial Intelligence (Generative AI), is dedicated to machines’ proficiency in generating content, spanning text, images, music, or even code. Remarkably, models like GPT-3 showcase their ability to generate human-like text and grasp contextual nuances. Here’s how Generative AI is transforming data-driven decision making:
Natural Language Processing (NLP):
Generative AI excels at understanding and generating natural language. This capability is invaluable for analyzing unstructured text data, such as customer reviews, social media comments, or survey responses. By extracting insights from text data, organizations can understand sentiment, identify emerging trends, and respond to customer feedback more effectively.
Data Augmentation:
Generative AI can be used to generate synthetic data. This is particularly useful in situations where real data is scarce or sensitive. By generating additional data points, organizations can improve the accuracy and robustness of their machine learning models.
Content Generation:
Businesses can leverage Generative AI to automate content generation for marketing, customer support, and other communication channels. This not only saves time and resources but also ensures consistent and high-quality content.
Predictive Analytics:
Generative AI models can help predict future trends based on historical data and current market conditions. By generating forecasts and scenario analyses, organizations can make proactive decisions to stay ahead of the curve.
Personalization and Recommendation:
Generative AI can be used to create personalized recommendations for customers, enhancing the customer experience. For example, e-commerce platforms can use AI-generated product recommendations to increase sales and customer engagement.
Leading Companies in Generative AI: Transforming Data-Driven Decision Making
OpenAI:
GPT-3, OpenAI’s Generative Pre-trained Transformer 3 (GPT-3) is a breakthrough model known for its natural language generation capabilities. It has been used in content generation, chatbots, and even code generation. GPT-3’s versatility has opened doors to a wide range of applications, from drafting articles and answering questions to generating creative writing and assisting developers with coding tasks.Google:
BERT (Bidirectional Encoder Representations from Transformers), Google’s BERT model revolutionized natural language processing tasks by understanding context in a bidirectional manner. It’s used in search engine algorithms to provide more accurate search results. BERT has greatly improved the way search engines interpret user queries, delivering more relevant and context-aware search results, which is crucial in today’s information-rich digital landscape.Microsoft:
Microsoft Azure Cognitive Services, Microsoft offers a suite of services powered by Generative AI, including language understanding, text analysis, and speech recognition, which are used for various data-driven applications, including sentiment analysis and chatbots. These services empower businesses to build intelligent applications that can interact with users through natural language, understand sentiment, and process text data efficiently.Salesforce:
Einstein Language, Salesforce’s Einstein platform utilizes AI, including Generative AI, to enhance customer relationship management. It can analyze customer interactions to provide recommendations and insights. Einstein Language enables organizations to extract valuable insights from customer interactions, driving better engagement and informed decision-making.IBM:
IBM Watson employs Generative AI for natural language understanding and generation. It’s used in healthcare for diagnosing diseases, in finance for risk assessment, and in chatbots for customer service. Watson’s ability to comprehend complex medical data, assess financial risks, and provide human-like responses in customer support has made it a valuable tool across multiple industries.Facebook:
PyTorch, Facebook’s open-source deep learning framework, PyTorch, is widely used for developing Generative AI models. Researchers and developers use PyTorch for various data-driven applications, including image and text generation. PyTorch’s flexibility and extensive community support have made it a preferred platform for innovators in the field, fostering the development of cutting-edge Generative AI applications.GeekyAnts:
In the realm of digital transformation, where innovation and agility are the driving forces, GeekyAnts emerges as a visionary company at the forefront of harnessing Generative AI to reshape the future of technology. Established as a dynamic player in the software development arena, GeekyAnts has garnered attention for its groundbreaking work in Generative AI and its profound impact on digital transformation efforts.Adobe:
Adobe Sensei, Adobe’s AI platform, Sensei, incorporates Generative AI to assist creative professionals in content generation, image manipulation, and design optimization. Sensei’s intelligent features simplify the creative process, making it easier for designers and content creators to produce high-quality content with efficiency and precision.Uber:
Generative Adversarial Networks (GANs), Uber employs GANs, a subset of Generative AI, for improving self-driving car simulations. GANs generate synthetic data to enhance the realism of training simulations. By using GANs, Uber can create highly realistic virtual environments for testing and training autonomous vehicles, accelerating the development of safe and reliable self-driving technology.
Netflix:
Content Recommendation, Netflix utilizes Generative AI algorithms to personalize content recommendations for its viewers, enhancing user engagement and satisfaction. These algorithms analyze user preferences and viewing habits to suggest content tailored to individual tastes, ensuring that viewers discover and enjoy content that resonates with them.
Also Read: “The Future Landscape of Generative AI-Driven Apps“.
Challenges and Considerations
While Generative AI holds immense promise for data-driven decision making, it also comes with challenges and considerations:
Ethical and Bias Concerns:
AI models can inherit biases from the data they are trained on, potentially leading to biased decision-making. Organizations must be vigilant in addressing these biases and ensuring fairness in their AI applications.
Data Privacy:
Generating synthetic data raises concerns about data privacy and security. Organizations must implement robust data protection measures and comply with relevant regulations.
Model Training and Maintenance:
Generative AI models require significant computational resources for training and ongoing maintenance. Organizations need to invest in infrastructure and expertise to deploy and manage these models effectively.
Interpretable AI:
Understanding the decisions made by AI models is crucial for trust and accountability. Developing methods to interpret and explain Generative AI outputs is an ongoing research challenge.
Conclusion
Data-driven decision making is central to the success of modern organizations undergoing digital transformation. Generative AI is emerging as a key enabler of this process, offering powerful tools for data analysis, content generation, and predictive insights. However, it is essential for organizations to approach Generative AI with a thoughtful and ethical mindset, addressing issues of bias, privacy, and transparency. By harnessing the potential of Generative AI, businesses can make more informed decisions, gain a competitive advantage, and drive innovation in the ever-evolving digital landscape.