Generative AI vs Traditional AI – these two approaches are at the forefront of artificial intelligence evolution. In this article, we’ll dive into the key differences between Generative AI and Traditional AI, highlighting how Generative AI’s data-driven creativity and adaptability are transforming the field.
Artificial Intelligence (AI) has evolved significantly over the years, and one of the most notable advancements is the emergence of Generative AI. While Traditional AI and Generative AI both fall under the broader umbrella of AI, they have distinct differences in terms of their underlying principles, capabilities, and applications. In this blog, we’ll explore these differences in greater detail to gain a deeper understanding of how each approach works and where they are commonly applied.
Traditional AI: Rule-Based and Expert-Driven
Traditional AI, also known as Classical AI or Symbolic AI, is the earlier paradigm of artificial intelligence that relies on predefined rules, logic, and expert knowledge. In Traditional AI systems, human experts play a crucial role in encoding explicit rules and knowledge into the software. These systems follow a deterministic approach, where the AI makes decisions based on explicit instructions and data patterns.
Key Characteristics of Traditional AI:
Some of the key characteristics of Traditional AI are as follows:
Rule-Based Systems:
Deterministic Decision-Making:
Traditional AI systems follow predetermined rules and logic, leading to deterministic decision-making. If specific conditions are met, the system will always produce the same output, making it predictable but potentially inflexible in dynamic situations.
Expert-Dependent:
The creation and maintenance of rule-based systems heavily depend on domain experts. These experts must possess deep knowledge of the problem domain and carefully craft rules to cover all relevant scenarios.
Explicit Knowledge Representation:
In Traditional AI, knowledge is explicitly represented in the form of rules. These rules are often written in human-readable language and can be reviewed and modified by experts when needed.
Limited Adaptability:
Rigidity in Changing Environments:
Traditional AI systems are not well-suited for tasks in environments where conditions are constantly changing. Since they rely on fixed rules, they may struggle to adapt to new or unforeseen circumstances.
Inability to Learn from Data:
Unlike Generative AI, which can learn and adapt from new data, Traditional AI systems do not possess the capability to learn from experience or data. They remain static unless manually updated.
Challenges with Unstructured Data:
Dealing with unstructured or noisy data, such as natural language text or real-world sensor data, can be challenging for Traditional AI. Extracting meaningful information from unstructured data often requires extensive rule engineering.
Lack of Creativity:
Limited Problem-Solving Scopes:
Traditional AI systems are designed for specific, well-defined problem domains. They lack the ability to think creatively or tackle problems outside their predefined scope.
Absence of Novelty:
Since Traditional AI follows pre-established rules and patterns, it cannot generate novel or unexpected solutions to problems. It relies on human-defined strategies and knowledge.
Not Suitable for Creative Tasks:
Tasks that involve artistic creativity, ideation, or generating innovative solutions typically fall outside the capabilities of Traditional AI. These systems lack the imagination required for such endeavors.
Examples of Traditional AI
Let’s explore some key application areas where Traditional AI has made significant contributions:
Expert Systems:
Traditional AI shines in applications that demand domain-specific knowledge and strict rule-based decision-making. Expert systems, a prominent example, leverage human expertise to create knowledge bases and rule sets that guide intelligent decision-making. These systems find applications in fields such as medicine and finance, where they assist professionals in diagnosing medical conditions, recommending treatments, or providing financial advice. Expert systems serve as valuable tools that complement human expertise and enhance decision accuracy.
Game Playing:
In the realm of game playing, Traditional AI has left its mark through remarkable achievements. Notably, IBM’s Deep Blue made headlines with its ability to play chess at a world-class level. Traditional AI algorithms underpin the strategies employed by Deep Blue and other chess engines, allowing them to explore vast move possibilities, evaluate board positions, and make precise decisions. Additionally, Traditional AI has been employed in the development of rule-based video game NPCs (non-player characters) that interact with players, adding strategic depth and challenge to gaming experiences.
Industrial Automation:
In the realm of industrial automation, Traditional AI plays a crucial role in optimizing manufacturing and process control. Tasks like quality control, where precise measurements and consistent decision-making are vital, benefit from Traditional AI systems. These systems can monitor production lines, detect defects in real-time, and make immediate decisions to maintain product quality. Furthermore, Traditional AI algorithms are used in optimizing production processes to enhance efficiency and reduce resource wastage.
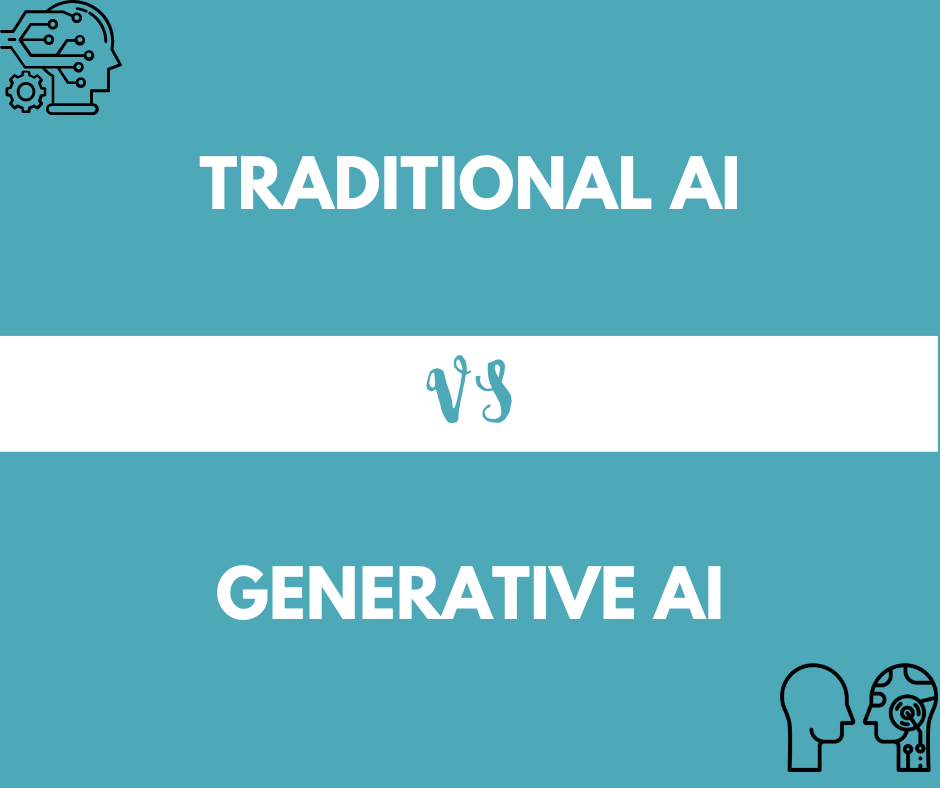
Generative AI: Learning from Data
Generative AI represents a paradigm shift in artificial intelligence. Instead of relying on explicit rules and human knowledge, Generative AI systems are trained on vast datasets using machine learning techniques, particularly deep learning. These systems learn patterns, representations, and probabilistic models from the data and use this knowledge to generate content or make decisions.
Key Characteristics of Generative AI:
Some of the key characteristics of Generative AI:
Data-Driven:
Generative AI systems learn from data, which allows them to generalize and perform well on a wide range of tasks without explicit rule-setting. By analyzing vast datasets, these models capture underlying patterns and structures, enabling them to make informed decisions and generate content based on learned information.
Creativity and Novelty:
Generative AI models possess a remarkable capacity for creativity and novelty. They can go beyond merely mimicking existing data and generate entirely new and innovative content. Whether it’s generating human-like text, producing unique artwork, or composing music that has never been heard before, Generative AI pushes the boundaries of creative expression.
Adaptability:
One of the key strengths of Generative AI is its adaptability. These systems can continuously learn and evolve as they encounter new data and changing contexts. This adaptability makes them well-suited for dynamic and unpredictable environments where traditional rule-based approaches might fall short. Generative AI models can adjust their outputs and responses to match the evolving nature of their tasks.
Natural Language Understanding:
Many Generative AI models, particularly those in the field of Natural Language Processing (NLP), exhibit an impressive understanding of human language. They can comprehend the nuances of context, tone, and semantics in text data, enabling them to generate coherent and contextually relevant responses in human-like language.
Multimodal Capabilities:
Beyond text, Generative AI has extended its reach to other modalities such as images and audio. Some models can generate realistic images from textual descriptions, apply artistic styles to photos, or even generate speech that closely resembles human voices. These multimodal capabilities open up diverse application possibilities.
Transfer Learning:
Generative AI models are often pretrained on massive datasets and then fine-tuned for specific tasks. This transfer learning approach allows them to leverage knowledge gained from one domain to perform well in related domains with limited additional training. It accelerates the development of AI solutions and facilitates a wide range of applications.
Human-AI Collaboration:
Generative AI is increasingly being used to augment human creativity and productivity. Rather than replacing humans, these systems collaborate with them. Content creators, artists, writers, and musicians, among others, use Generative AI as a creative tool, harnessing its capabilities to enhance their work.
Ethical Considerations:
The growing power and creativity of Generative AI raise important ethical considerations. As these systems become capable of generating highly convincing fake content, there are concerns about misinformation, privacy, and the responsible use of AI-generated materials. Ethical guidelines and safeguards are crucial to address these challenges.
Examples of Generative AI
Some of the examples for Generative AI:
Natural Language Processing (NLP):
Generative AI models like GPT-3 have revolutionized NLP tasks, including language translation, text generation, and chatbots. These models can translate text between languages with remarkable accuracy, produce coherent and contextually relevant text based on prompts, and power conversational AI systems that interact with users in a human-like manner.
Computer Vision:
Generative models can generate and manipulate images, making them valuable for image synthesis, style transfer, and image-to-image translation. They can create realistic images from textual descriptions, apply artistic styles to photographs, and even transform photographs in ways that were previously only possible through manual editing.
Art and Creativity:
Generative AI has been applied to create art, music, and literature, blurring the lines between human and machine creativity. Artists and musicians use AI-generated content as a source of inspiration, collaborating with AI to produce artworks, compositions, and literary pieces that push the boundaries of artistic expression.
Content Generation:
Content creators use Generative AI to automate content production, such as generating news articles, product descriptions, and marketing content. These systems can quickly generate high-quality content at scale, freeing human creators to focus on higher-level creative and strategic tasks while maintaining a consistent content output.
Challenges and Future Directions
While Generative AI offers exciting possibilities, it also comes with its own set of challenges, including ethical concerns, biases in training data, and the potential for misuse. Striking the right balance between automation and human oversight remains a critical concern.
In the future, we can expect Generative AI to continue advancing, enabling more creative and adaptive applications across various domains. Additionally, research in explainability and fairness will be crucial to ensure that AI systems are transparent and unbiased.
Also Read : ” How generative AI can help improve business“
Conclusion
In conclusion, Traditional AI and Generative AI represent two distinct approaches to artificial intelligence, with Traditional AI relying on predefined rules and expertise and Generative AI learning from data to generate creative outputs. Both have their strengths and weaknesses, and their suitability depends on the specific task and context. As AI technology continues to evolve, understanding the differences between these approaches is essential for making informed decisions about which AI techniques to leverage in various applications.